Picture your GIS software not only being knowledgeable but also continually learning, becoming more intelligent and adept at understanding the world around us. Welcome to the fascinating realm of GeoAI, where your maps don a superhero cape, guiding you through paths and predicting tomorrow’s traffic snarls.
GeoAI is the fusion of geospatial data with artificial intelligence, aimed at scrutinizing geographical information to make more precise predictions. Today, let’s delve into this captivating world of GeoAI.
Key Technologies
Who leads the charge in GeoAI? Currently, there are a few major players that stand out. Let’s take a closer look at some of them:
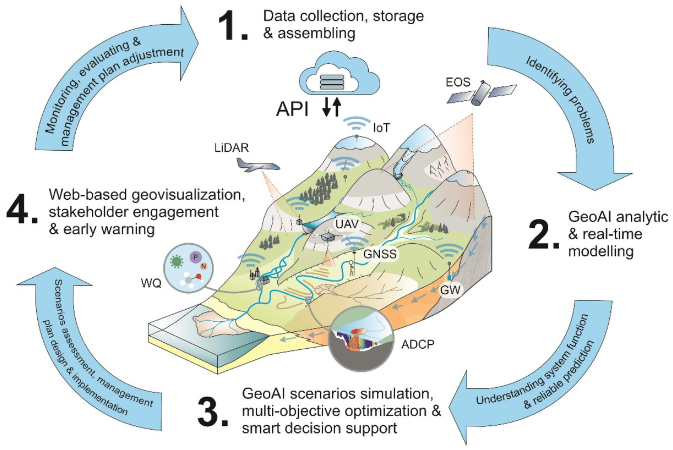
ESRI ArcGIS: A global leader in GIS technology, ArcGIS incorporates AI and machine learning for spatial analysis and predictive modeling. For examples, explore Esri’s YouTube GeoAI channel, where features like extraction and deep learning using the arcgis.learn module are showcased.
Google Earth Engine (GEE): GEE combines a massive catalog of satellite imagery and geospatial datasets, enabling planetary-scale analysis capabilities such as model training and regression prediction.
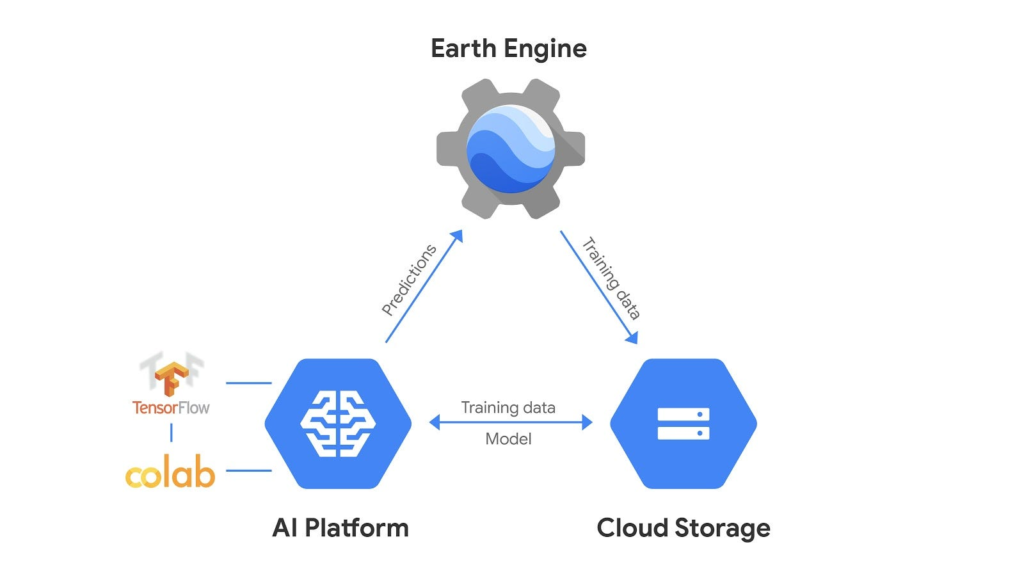
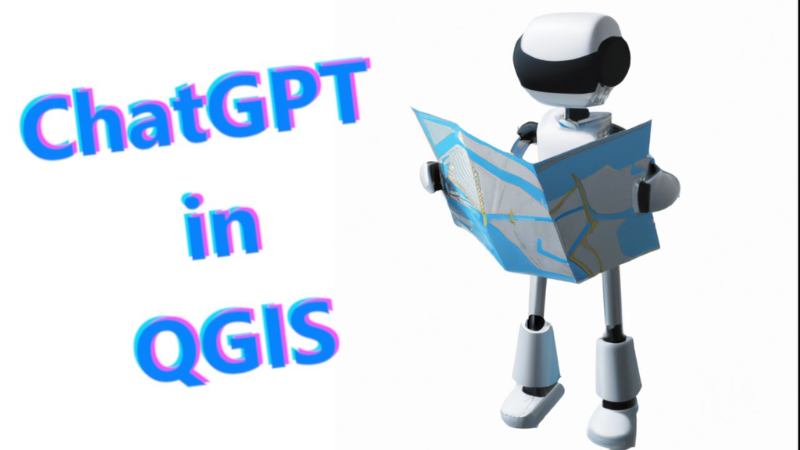
QGIS with AI plugins: Though a bit behind in AI capabilities, QGIS is catching up. AI plugins enable advanced geospatial data analysis, including clustering, segmentation, and deep neural networks.
While these tools are evolving and becoming more intelligent every day, it’s important to note that they are still in the growth stage.
Applications of GeoAI
GeoAI finds applications in various fields, including environmental monitoring, urban planning, disaster management, and transportation. Here are some key examples:
FLOOD PREDICTION: GeoAI models can predict flood-prone areas by analyzing terrain data and weather patterns, improving emergency response.
DEFORESTATION DETECTION: Using satellite imagery, GeoAI identifies changes in forest cover over time, aiding efforts to combat deforestation and preserve biodiversity.
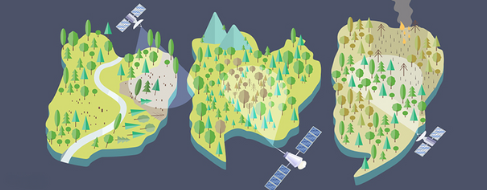
CITY HEAT MAPPING: GeoAI analyzes urban heat islands using satellite and aerial imagery, helping city planners design livable urban environments.
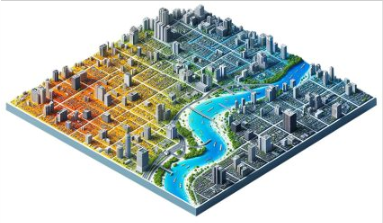
TRAFFIC FLOW OPTIMIZATION: GeoAI analyzes traffic patterns from sources like GPS and road sensors, contributing to traffic flow optimization and reducing congestion in cities.
These examples showcase GeoAI’s practical applications in addressing real-world challenges across different sectors.
Challenges and Limitations
In the field of GeoAI, its effectiveness can be limited by the quality and availability of geospatial data. Consider your data as a GIS analyst – is it complete, and how accurate is it? GeoAI faces challenges with sparse or outdated GIS data.
Another significant challenge is ensuring data privacy and security, given that geospatial data often contains sensitive information. Protecting data privacy becomes crucial.
Finally, the resource-intensiveness of GeoAI models can pose challenges in real-time decision-making scenarios. Processing large datasets in real time may strain the capabilities of computers running software like ArcGIS Pro.
What’s Next for GeoAI?
In the larger context, GeoAI stands at the crossroads of artificial intelligence and geography. Its power lies in converting vast and complex datasets into actionable insights, making our world more comprehensible and manageable.
Looking forward, the future of GeoAI appears promising. As technology matures and demand increases, unlocking GeoAI’s full potential will become key.
As we navigate this exciting new world of GeoAI, let’s keep one foot in reality and one in the realm of possibility. And for now, perhaps it’s wise to keep a paper map in the glove box, just in case.