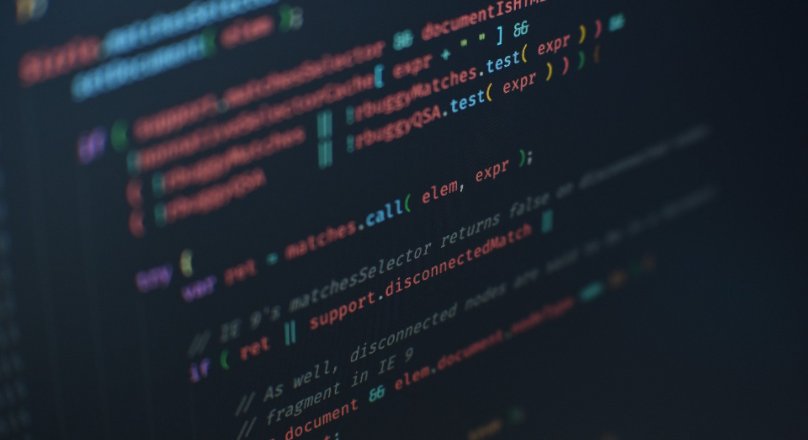
Introduction
Interactive maps have become indispensable in fields like urban planning, environmental science, and business analytics, transforming raw geospatial data into actionable insights. Python, with its flexibility, open-source ecosystem, and seamless integration with modern workflows, has emerged as a powerhouse for GIS web mapping. In this article, we explore five Python libraries that cater to diverse needs—from quick visualizations to enterprise-grade dashboards—and provide code examples to kickstart your next project.
Criteria for Selection
We evaluated libraries based on:
- Ease of Use: Accessibility for beginners.
- Customization: Flexibility to tailor maps.
- Integration: Compatibility with web frameworks.
- Interactivity: Support for real-time features.
- Community Support: Documentation and updates.
1. Folium: Simple Maps for Beginners
Overview: Built on Leaflet.js, Folium is ideal for newcomers.
Key Features:
- Intuitive syntax for markers, popups, and choropleth maps.
- Export maps to HTML for easy sharing.
Use Case: Visualize earthquake data in Jupyter notebooks.
Code Snippet:
import folium
map = folium.Map(location=[37.7749, -122.4194], zoom_start=12)
folium.Marker([37.7749, -122.4194], tooltip="San Francisco").add_to(map)
map.save("map.html")
2. Plotly Dash: Data-Driven Dashboards
Overview: A framework for building analytical web apps.
Key Features:
- Combines Plotly visualizations with Flask/React.
- Real-time updates via dropdowns and sliders.
Use Case: Track logistics routes in a corporate dashboard.
Code Snippet:
import dash
import dash_core_components as dcc
import plotly.express as px
app = dash.Dash()
app.layout = dcc.Graph(figure=px.scatter_mapbox(lat=[40.7128], lon=[-74.0060]))
app.run_server(debug=True)
3. Geemap: Satellite Imagery Powerhouse
Overview: Bridges Python with Google Earth Engine (GEE).
Key Features:
- Access petabytes of satellite imagery.
- Create time-series animations.
Use Case: Monitor deforestation in the Amazon.
Code Snippet:
import geemap
Map = geemap.Map()
Map.add_basemap("SATELLITE")
Map
4. PyDeck: 3D Mapping for Big Data
Overview: Uber’s high-performance library leveraging Deck.gl.
Key Features:
- GPU-accelerated rendering for massive datasets.
- 3D visualizations like point clouds and hexbins.
Use Case: Visualize global ride-sharing patterns.
Code Snippet:
import pydeck as pdk
layer = pdk.Layer("ScatterplotLayer", data={"lat": [34.0522], "lon": [-118.2437]})
view_state = pdk.ViewState(latitude=34.0522, longitude=-118.2437, zoom=10)
pdk.Deck(layers=[layer], initial_view_state=view_state).to_html("3d_map.html")
5. ipyleaflet: Jupyter-Friendly Mapping
Overview: Interactive maps within Jupyter notebooks.
Key Features:
- Widget support for WMS/GeoJSON layers.
- Integrates with Jupyter Lab/Notebook.
Use Case: Collaborative climate research.
Code Snippet:
from ipyleaflet import Map, TileLayer
m = Map(center=(51.5074, -0.1278), zoom=10)
m.add_layer(TileLayer(url="https://{s}.tile.openstreetmap.org/{z}/{x}/{y}.png"))
m
Comparison Table
Library | Best For | Learning Curve | Customization |
---|---|---|---|
Folium | Quick, shareable maps | Low | Medium |
Plotly Dash | Data-driven dashboards | Medium | High |
Geemap | Satellite/remote sensing | Medium | High |
PyDeck | Big data/3D visualization | High | High |
ipyleaflet | Jupyter-based projects | Low | Medium |
Tips for Choosing the Right Library
- Beginners: Start with Folium or ipyleaflet.
- Enterprise Apps: Use Plotly Dash or PyDeck.
- Satellite Data: Leverage Geemap.
Conclusion
From Folium’s simplicity to PyDeck’s 3D prowess, each library offers unique strengths. Whether you’re tracking shipping routes or analyzing deforestation, Python’s ecosystem has a tool for you. Experiment with these libraries and explore their official documentation to unlock their full potentia