In the realm of remote sensing, the Leaf-Water Stress Index (LSWI) and the Moisture Stress Index (MSI) stand out as key tools for assessing vegetation water stress and soil moisture, respectively. These indices play a crucial role in mapping and monitoring various environmental factors.
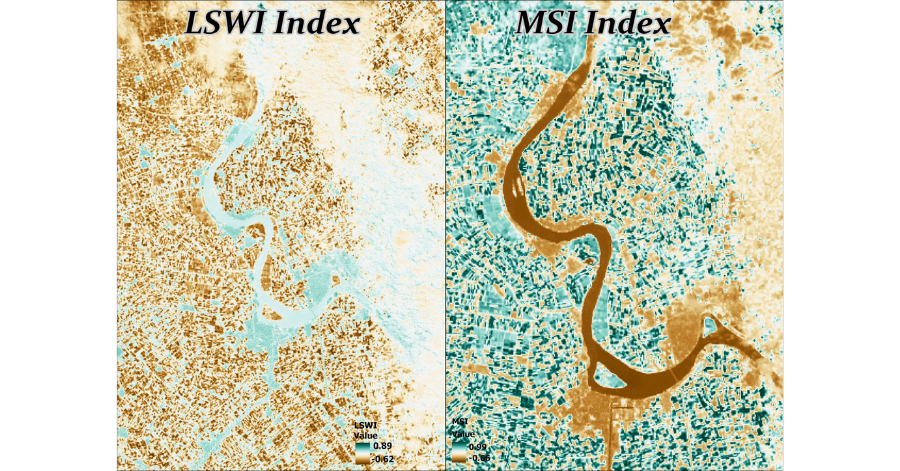
Understanding LSWI and MSI
LSWI, or Leaf-Water Stress Index, is computed by taking the normalized difference between reflectance in the near-infrared (NIR) and red bands, normalized by their sum. The resulting values range from -1 to 1, with higher values indicating lower water stress in vegetation. On the other hand, MSI, the Moisture Stress Index, gauges soil moisture by calculating the ratio of NIR band reflectance to shortwave infrared (SWIR) band reflectance. MSI values range from 0 to 1, with higher values signifying higher soil moisture levels.
Key Differences in Calculation
The primary distinction between LSWI and MSI lies in their respective formulas. While LSWI employs the difference between NIR and red bands, MSI relies on the ratio of NIR and SWIR bands. This dissimilarity makes LSWI more attuned to changes in leaf water content, while MSI is more responsive to alterations in soil moisture.
Applications in Mapping and Monitoring
Both LSWI and MSI find applications in monitoring vegetation health and productivity. The choice between these indices depends on the specific characteristics of the study area and the research question at hand. These indices, with their ability to quantify changes in vegetation and soil conditions, are vital in mapping land surface water index, detecting landsat, and studying the effects of drought.
Utilizing Satellite Data and Advanced Techniques
These indices make use of satellite data, including Sentinel-2 MSI and MODIS, to achieve high spatial resolution in mapping. The inclusion of spectral bands and normalized difference vegetation index (NDVI) enhances their accuracy. Researchers employ various satellite sensors, such as Landsat, to develop new methods for mapping land cover types and water bodies.
Case Study: Mapping Paddy Rice in Northeast China
A notable case study conducted in northeast China demonstrates the feasibility of using a new method incorporating Sentinel-2 MSI images and multi-temporal satellite data. This approach, developed using advanced techniques, exhibited high accuracy in mapping paddy rice fields. The study utilized the strengths of Sentinel-2 MSI satellite data, highlighting its efficiency in detecting and monitoring drought effects in the region.
In conclusion, the application of LSWI and MSI in remote sensing is wide-ranging, from mapping surface water to studying the spatial distribution of surface features. Utilizing these indices, researchers continue to advance methodologies, contributing to the field of geoscience and remote sensing. The integration of high-resolution satellite data and advanced techniques ensures the precision and reliability of these indices in diverse research areas.